Recommendation systems in e-commerce
Recommendation systems have gained importance in recent years, and appear very promising for e-commerce, especially in connection with Big Data. By working with large amounts of data and using sophisticated algorithms, you can increase the conversions of online stores with modern recommendation systems. You are no doubt familiar with the classic 'customers who bought this item also bought…' or 'the following products may interest you…' notifications in online shopping. These tips, corresponding to the individual preferences of the user, result from considerable computing power and complicated algorithms. In e-commerce, recommendation systems are already incorporated, but other sectors also benefit from continuously improving algorithms and technologies.
Sell and market your craft with our intuitive online store builder.
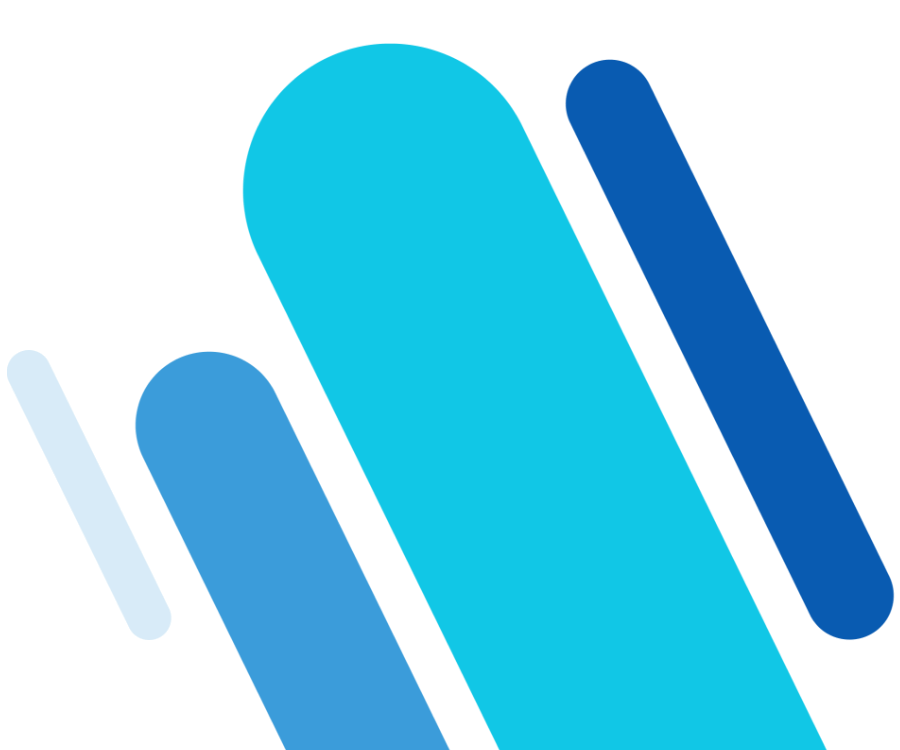
What are recommendation systems?
A recommendation system (also known as 'recommender system', 'recommendation engine', or 'recommendation platform') is, according to the definition, an information filtering system that attempts to predict the 'rating' or 'preference' that a user might give to an item or product. It analyzes past behavior and previous orders, and automatically searches for similar and interesting products for the user. The field of application for recommendation systems is diverse. You will recognize recommendations from web stores, streaming services, and online publications – wherever large amounts of objects are, whether they are books, clothes, or movies – but only a small amount has any relevance to the user. Considering the amount of data and possible search paths, recommendations help by selecting a small amount in advance from the confusing selection and presenting it to the user.
Advantages of recommendation systems
These systems should make searching a lot easier for the user. Instead of clicking through lots of offers and pages to find the right product, this pre-selection aims to exclude irrelevant and uninteresting offers so that the ones displayed are the ones most suited to the user.
Operators also hope for a positive effect, such as an increase in visitor numbers in the content sector or an increase in sales in e-commerce. In online stores, suitable suggestions should ideally lead to bigger shopping carts, which then significantly increase margins.
But calculating using algorithms also comes with its disadvantages. Often personal, human components are missing when it comes to the suggested selection. Even sophisticated calculations can read simple human behaviors wrong and are more likely to leave the user shaking their head rather than impress them. For example, Amazon might display a glass cutter, which many craftsmen need for their daily work, but the selection also shows balaclavas and other accessories more suited to burglars.
How do recommendation systems work?
Recommendation services are always based on sets of data. Depending on the nature of this data set, a distinction is made between different types of systems. These are typically content-based and collaborative systems. Furthermore, there are also context-sensitive recommendation services, as well as those that include the chronological sequence or demographic user data in the analysis.
Content-based recommendation systems
Content-based recommendation systems suggest objects or content similar to what the user has already searched for, viewed, bought, or rated highly. The system must be able to establish a similarity between objects. This is done through content analysis. For music streaming services, for example, the system analyzes a piece of music (e.g. the structure of the music) to find pieces that have a similar bass track.
Collaborative recommendation systems
When it comes to collaborative methods, the suggestions are based on users with similar rating behavior. If they showed a lot of interest in a particular object in the past, the system continues to suggest it. Information or knowledge about the object itself is not necessary at this point. Amazon, for example, uses this method extensively.
Different prediction methods
Recommendation services use different learning methods. In general, either the memory-based or the model-based method is used. The memory-based method uses all stored evaluation data and calculates the similarity between users or objects. The result is the base, which enables user-object combinations to be predicted. Model-based recommendation services, on the other hand, work with the principles of machine learning. Based on the data, the system can create a mathematical model that can be used to predict the user’s interest in a particular product.
Examples of well-known recommendation systems
Recommendation systems can be found in many sectors and branches. The three most important are large streaming services such as Spotify or Netflix, classic e-commerce providers such as Amazon, and special recommendation systems for content-based advertising.
Streaming services: Netflix and Spotify’s recommendation services
The video streaming service, Netflix, first integrated a new recommendation system into its platform at the beginning of 2016. The algorithm displays suggestions depending on each Netflix user’s personal taste in movies and series. These algorithms, however, don’t take demographic data into account, such as age and gender. It’s simply the collected data that is used to decide on which suggestions to display. When the user sets up their account, they are asked to reveal their favorite movies and series. Questions need to be answered such as 'what has the customer previously seen?' and 'how did they rate it?'. By comparing all customers based on their preferences and ratings, the platform can make accurate suggestions. There used to be problems every time the service was introduced in a new country. This is because there was no previous data to calculate any recommendations from. The new algorithm works with transnational customer groups. In this context, country-specific and region-specific tendencies are still included. The music streaming service, Spotify, has been working with personal recommendations for a long time. The service compiles a list of songs each week that potentially match the user’s taste. Your Daily Mix playlist is automatically created by algorithms. These playlists are partly self-generated playlists from other users that the user creates themselves and partly Spotify attempting to build an accurate profile depending on the user’s tastes. The service works with extremely narrow genre definitions. A software is used additionally that analyzes articles and texts on blogs and magazines to classify artists as accurately as possible. The recommendation service also recognizes so-called genre anomalies, which do not fit into the overall profile because the user maybe decided to play the song on a whim. Spotify doesn’t include these songs in the personalized playlist.
E-commerce: product recommendations on Amazon and similar sites
In e-commerce, product recommendations are basically based on classic cross-selling: users are shown matching or supplementary products. Amazon is well ahead regarding product recommendations. The market leader has a huge pool of user-generated data available. Early on, the e-commerce giant recognized the fact that with the right product recommendations, the customer’s shopping carts fill up more quickly. In the meantime, you can find up to five different types of product recommendations at different points in the purchasing process:
- 'Customers who viewed this item also viewed'
- 'Customers who bought this item also bought'
- 'Frequently bought together'
- 'What do customers buy after viewing this item?'
- 'Your recently viewed items and featured recommendations'
Amazingly, Amazon released its deep-learning software DSSTNE as open source at the beginning of the year – the software is the basis for recommendations on Amazon. Basically, there’s a trend towards more in-depth recommendation systems in e-commerce. In addition to the possibility of displaying 'Popular items', more and more companies rely on highly personalized recommendations. As a rule, several recommendation strategies are brought together: purchasing interests, popular items, and other factors, such as product availability and price changes, are automatically included.
Content recommendation systems from Outbrain and Revcontent
What works on Netflix with movies and series, and on Amazon with cameras and books, is also successful in native advertising. Most people are familiar with notifications on online magazine pages such as 'this could interest you' or 'similar items', with content from external sites. Here, recommendation technologies are part of native advertising strategies. Outbrain and Revcontent are well-known native ad providers in the US.
Software for recommendation systems in e-commerce
In e-commerce, recommendation systems are a particularly important topic. This is because online stores have the possibility to increase their conversion rate through suitable recommendations and generate more sales. Many shop systems have integrated standard features for product recommendations. This allows a solid analysis and calculation– but the best way is to use a special software solution. Various providers offer companies SaaS (software-as-a-service) solutions. Well-known solutions in the US include Certona and Barilliance. Most providers promise software solutions, which are individually tailored and capable of self-learning, as recommendation services based on their own personalization technology (model-based method). The great advantage of SaaS solutions is that they significantly reduce the time and effort required for implementation. Store owners don’t have to invest in hardware or software. The mostly cloud-based solutions also feature a large range of functions. The software solutions take on three important steps: database tracking, feature engineering, and data processing or analyzing at the end.
Tracking databases
To be able to analyze data, you have to collect it first. This can be done through classic tracking methods for most software solutions. Tracking includes relevant data on the location, shopping cart, date and time, behavior, and usually the completely traceable customer journey. The program collects all this information and keeps it in a database.
Feature engineering
When it comes to feature engineering, the goal is to filter out features (i.e. characteristics, properties) from the database. These features can be of a different nature, such as the time of the visit and its duration, the distances between actions, and many more. However, few features are of any relevance to the predictions later on. The challenge that the system faces, is to precisely identify these important features. For this purpose, the system needs to find the characteristics that have a significant influence on the purchasing behavior, and ultimately, the purchase decision. The individual composition of the features varies according to the store, so an intelligent analysis is required.
Processing and analyzing data
Based on the features defined for the online store i.e. the relevant characteristics and properties, the system can now calculate predictions for product recommendations. Creating these prognosis models requires a huge amount of computing power, and sometimes takes several hours. The system saves the models, which then serve as a basis for calculating recommendations. Every store visitor receives up-to-date tips and recommendations tailored to them.
Conclusion
Personalization is becoming increasingly important in online marketing. This is not only due to the fact that companies feel under pressure from their competition and have to constantly strive to stand out from the crowd, but also because of users’ changed perceptions. Today’s users are able to identify advertisements more quickly and ignore them. If you are, however, able to attract attention by providing customized and relevant information, and by addressing users directly, the chance of conversion is much higher. The same is true for recommendation systems, which are becoming more sensitive and accurate. Finding the right strategy and reaching potential customers can have a positive impact on sales and the success of a company in e-commerce.